CS301_Proj10
Introduction
For your final CS 301 project, you’re going to analyze the whole
world!
Specifically, you’re going to study various statistics for 174
countries, answering questions such as: what is the correlation
between a country’s literacy rate and GDP?
To start, download test.py
and expected.html
. You’ll also need to
download lint.py
(see linter documentation under “Testing” below).
Do not download any data files manually (you must write Python code to
download these automatically). You’ll do all your work in a
main.ipynb
.
Data
For this project, you’ll be using one large JSON file with statistics
about 174 countries adapted from
here.
and you will also extract data from a snapshot of
this page.
First check these resources:
Some of the columns require a little extra explanation:
- area: measured in square miles
- coastline: ratio of coast to area
- birth-rate: births per 1000 people per year
- death-rate: deaths per 1000 people per year
- infant-mortality: per 1000 births
- literacy: (out of 100%)
- phones: number of phone per 1000 people
import pandas as pd
import requests,json
from bs4 import BeautifulSoup
import os
from pandas import DataFrame, Series
from math import radians, cos, sin, asin, sqrt
import numpy as np
import matplotlib.pyplot as plt
def download(filename, url):
if os.path.exists(filename):
return (str(filename) + " already exists!")
else:
r = requests.get(url)
r.raise_for_status()
data = r.text
f = open(filename, "w", encoding="utf-8")
f.write(data)
f.close()
return (str(filename) + " created!")
download("countries.json","https://raw.githubusercontent.com/tylerharter/caraza-harter-com/master/tyler/cs301/fall19/data/countries.json")
'countries.json already exists!'
countries = pd.read_json('countries.json')
countries
|
area |
birth-rate |
coastline |
continent |
country |
death-rate |
gdp-per-capita |
infant-mortality |
literacy |
net-migration |
phones |
population |
region |
0 |
756950 |
15.23 |
0.85 |
South America |
Chile |
5.81 |
9900 |
8.80 |
96,2 |
0.00 |
213,0 |
16134219 |
LATIN AMER. & CARIB |
1 |
665 |
17.80 |
24.21 |
Asia |
Bahrain |
4.14 |
16900 |
17.27 |
89,1 |
1.05 |
281,3 |
698585 |
NEAR EAST |
2 |
22966 |
28.84 |
1.68 |
North America |
Belize |
5.72 |
4900 |
25.69 |
94,1 |
0.00 |
115,7 |
287730 |
LATIN AMER. & CARIB |
3 |
582650 |
39.72 |
0.09 |
Africa |
Kenya |
14.02 |
1000 |
61.47 |
85,1 |
-0.10 |
8,1 |
34707817 |
SUB-SAHARAN AFRICA |
4 |
11854 |
33.05 |
3.12 |
Australia |
Marshall Islands |
4.78 |
1600 |
29.45 |
93,7 |
-6.04 |
91,2 |
60422 |
OCEANIA |
5 |
111370 |
44.77 |
0.52 |
Africa |
Liberia |
23.10 |
1000 |
128.87 |
57,5 |
0.00 |
2,3 |
3042004 |
SUB-SAHARAN AFRICA |
6 |
504782 |
10.06 |
0.98 |
Europe |
Spain |
9.72 |
22000 |
4.42 |
97,9 |
0.99 |
453,5 |
40397842 |
WESTERN EUROPE |
7 |
65610 |
15.51 |
2.04 |
Asia |
Sri Lanka |
6.52 |
3700 |
14.35 |
92,3 |
-1.31 |
61,5 |
20222240 |
ASIA (EX. NEAR EAST) |
8 |
212460 |
36.24 |
0.98 |
Asia |
Oman |
3.81 |
13100 |
19.51 |
75,8 |
0.28 |
85,5 |
3102229 |
NEAR EAST |
9 |
316 |
10.22 |
62.28 |
Europe |
Malta |
8.10 |
17700 |
3.89 |
92,8 |
2.07 |
505,0 |
400214 |
WESTERN EUROPE |
10 |
185180 |
27.76 |
0.10 |
Asia |
Syria |
4.81 |
3300 |
29.53 |
76,9 |
0.00 |
153,8 |
18881361 |
NEAR EAST |
11 |
1759540 |
26.49 |
0.10 |
Africa |
Libya |
3.48 |
6400 |
24.60 |
82,6 |
0.00 |
127,1 |
5900754 |
NORTHERN AFRICA |
12 |
752614 |
41.00 |
0.00 |
Africa |
Zambia |
19.93 |
800 |
88.29 |
80,6 |
0.00 |
8,2 |
11502010 |
SUB-SAHARAN AFRICA |
13 |
10991 |
20.82 |
9.30 |
North America |
Jamaica |
6.52 |
3900 |
12.36 |
87,9 |
-4.92 |
124,0 |
2758124 |
LATIN AMER. & CARIB |
14 |
447400 |
26.36 |
0.00 |
Asia |
Uzbekistan |
7.84 |
1700 |
71.10 |
99,3 |
-1.72 |
62,9 |
27307134 |
C.W. OF IND. STATES |
15 |
61 |
10.02 |
0.00 |
Europe |
San Marino |
8.17 |
34600 |
5.73 |
96,0 |
10.98 |
704,3 |
29251 |
WESTERN EUROPE |
16 |
488100 |
27.61 |
0.00 |
Asia |
Turkmenistan |
8.60 |
5800 |
73.08 |
98,0 |
-0.86 |
74,6 |
5042920 |
C.W. OF IND. STATES |
17 |
33843 |
15.70 |
0.00 |
Europe |
Moldova |
12.64 |
1800 |
40.42 |
99,1 |
-0.26 |
208,1 |
4466706 |
C.W. OF IND. STATES |
18 |
28748 |
15.11 |
1.26 |
Europe |
Albania |
5.22 |
4500 |
21.52 |
86,5 |
-4.93 |
71,2 |
3581655 |
EASTERN EUROPE |
19 |
53 |
11.40 |
194.34 |
North America |
Bermuda |
7.74 |
36000 |
8.53 |
98,0 |
2.49 |
851,4 |
65773 |
NORTHERN AMERICA |
20 |
803940 |
29.74 |
0.13 |
Asia |
Pakistan |
8.23 |
2100 |
72.44 |
45,7 |
-2.77 |
31,8 |
165803560 |
ASIA (EX. NEAR EAST) |
21 |
30528 |
10.38 |
0.22 |
Europe |
Belgium |
10.27 |
29100 |
4.68 |
98,0 |
1.23 |
462,6 |
10379067 |
WESTERN EUROPE |
22 |
47000 |
33.65 |
0.00 |
Asia |
Bhutan |
12.70 |
1300 |
100.44 |
42,2 |
0.00 |
14,3 |
2279723 |
ASIA (EX. NEAR EAST) |
23 |
587040 |
41.41 |
0.82 |
Africa |
Madagascar |
11.11 |
800 |
76.83 |
68,9 |
0.00 |
3,6 |
18595469 |
SUB-SAHARAN AFRICA |
24 |
17363 |
27.41 |
0.00 |
Africa |
Swaziland |
29.74 |
4900 |
69.27 |
81,6 |
0.00 |
30,8 |
1136334 |
SUB-SAHARAN AFRICA |
25 |
4167 |
16.68 |
60.60 |
Australia |
French Polynesia |
4.69 |
17500 |
8.44 |
98,0 |
2.94 |
194,5 |
274578 |
OCEANIA |
26 |
64589 |
9.24 |
0.82 |
Europe |
Latvia |
13.66 |
10200 |
9.55 |
99,8 |
-2.23 |
321,4 |
2274735 |
BALTICS |
27 |
93030 |
9.72 |
0.00 |
Europe |
Hungary |
13.11 |
13900 |
8.57 |
99,4 |
0.86 |
336,2 |
9981334 |
EASTERN EUROPE |
28 |
693 |
9.34 |
27.85 |
Asia |
Singapore |
4.28 |
23700 |
2.29 |
92,5 |
11.53 |
411,4 |
4492150 |
ASIA (EX. NEAR EAST) |
29 |
160 |
10.21 |
0.00 |
Europe |
Liechtenstein |
7.18 |
25000 |
4.70 |
100,0 |
4.85 |
585,5 |
33987 |
WESTERN EUROPE |
... |
... |
... |
... |
... |
... |
... |
... |
... |
... |
... |
... |
... |
... |
144 |
267667 |
36.16 |
0.33 |
Africa |
Gabon |
12.25 |
5500 |
53.64 |
63,2 |
0.00 |
27,4 |
1424906 |
SUB-SAHARAN AFRICA |
145 |
13790 |
12.77 |
3.63 |
North America |
Puerto Rico |
7.65 |
16800 |
8.24 |
94,1 |
-1.46 |
283,1 |
3927188 |
LATIN AMER. & CARIB |
146 |
338145 |
10.45 |
0.37 |
Europe |
Finland |
9.86 |
27400 |
3.57 |
100,0 |
0.95 |
405,3 |
5231372 |
WESTERN EUROPE |
147 |
1240000 |
49.82 |
0.00 |
Africa |
Mali |
16.89 |
900 |
116.79 |
46,4 |
-0.33 |
6,4 |
11716829 |
SUB-SAHARAN AFRICA |
148 |
2170 |
36.93 |
15.67 |
Africa |
Comoros |
8.20 |
700 |
74.93 |
56,5 |
0.00 |
24,5 |
690948 |
SUB-SAHARAN AFRICA |
149 |
7686850 |
12.14 |
0.34 |
Australia |
Australia |
7.51 |
29000 |
4.69 |
100,0 |
3.98 |
565,5 |
20264082 |
OCEANIA |
150 |
825418 |
24.32 |
0.19 |
Africa |
Namibia |
18.86 |
7200 |
48.98 |
84,0 |
0.00 |
62,6 |
2044147 |
SUB-SAHARAN AFRICA |
151 |
181040 |
26.90 |
0.24 |
Asia |
Cambodia |
9.06 |
1900 |
71.48 |
69,4 |
0.00 |
2,6 |
13881427 |
ASIA (EX. NEAR EAST) |
152 |
236040 |
47.35 |
0.00 |
Africa |
Uganda |
12.24 |
1400 |
67.83 |
69,9 |
0.00 |
3,6 |
28195754 |
SUB-SAHARAN AFRICA |
153 |
945087 |
37.71 |
0.15 |
Africa |
Tanzania |
16.39 |
600 |
98.54 |
78,2 |
-2.06 |
4,0 |
37445392 |
SUB-SAHARAN AFRICA |
154 |
27830 |
42.22 |
0.00 |
Africa |
Burundi |
13.46 |
600 |
69.29 |
51,6 |
-0.06 |
3,4 |
8090068 |
SUB-SAHARAN AFRICA |
155 |
82880 |
18.96 |
1.59 |
Asia |
United Arab Emirates |
4.40 |
23200 |
14.51 |
77,9 |
1.03 |
475,3 |
2602713 |
NEAR EAST |
156 |
329750 |
22.86 |
1.42 |
Asia |
Malaysia |
5.05 |
9000 |
17.70 |
88,7 |
0.00 |
179,0 |
24385858 |
ASIA (EX. NEAR EAST) |
157 |
78866 |
9.02 |
0.00 |
Europe |
Czech Republic |
10.59 |
15700 |
3.93 |
99,9 |
0.97 |
314,3 |
10235455 |
EASTERN EUROPE |
158 |
514000 |
13.87 |
0.63 |
Asia |
Thailand |
7.04 |
7400 |
20.48 |
92,6 |
0.00 |
108,9 |
64631595 |
ASIA (EX. NEAR EAST) |
159 |
70280 |
14.45 |
2.06 |
Europe |
Ireland |
7.82 |
29600 |
5.39 |
98,0 |
4.99 |
500,5 |
4062235 |
WESTERN EUROPE |
160 |
18270 |
22.55 |
6.18 |
Australia |
Fiji |
5.65 |
5800 |
12.62 |
93,7 |
-3.14 |
112,6 |
905949 |
OCEANIA |
161 |
2717300 |
16.00 |
0.00 |
Asia |
Kazakhstan |
9.42 |
6300 |
29.21 |
98,4 |
-3.35 |
164,1 |
15233244 |
C.W. OF IND. STATES |
162 |
1564116 |
21.59 |
0.00 |
Asia |
Mongolia |
6.95 |
1800 |
53.79 |
97,8 |
0.00 |
55,1 |
2832224 |
ASIA (EX. NEAR EAST) |
163 |
198500 |
22.80 |
0.00 |
Asia |
Kyrgyzstan |
7.08 |
1600 |
35.64 |
97,0 |
-2.45 |
84,0 |
5213898 |
C.W. OF IND. STATES |
164 |
527970 |
42.89 |
0.36 |
Asia |
Yemen |
8.30 |
800 |
61.50 |
50,2 |
0.00 |
37,2 |
21456188 |
NEAR EAST |
165 |
9984670 |
10.78 |
2.02 |
North America |
Canada |
7.80 |
29800 |
4.75 |
97,0 |
5.96 |
552,2 |
33098932 |
NORTHERN AMERICA |
166 |
262 |
12.74 |
61.07 |
North America |
Cayman Islands |
4.89 |
35000 |
8.19 |
98,0 |
18.75 |
836,3 |
45436 |
LATIN AMER. & CARIB |
167 |
118480 |
43.13 |
0.00 |
Africa |
Malawi |
19.33 |
600 |
103.32 |
62,7 |
0.00 |
7,9 |
13013926 |
SUB-SAHARAN AFRICA |
168 |
86600 |
20.74 |
0.00 |
Europe |
Azerbaijan |
9.75 |
3400 |
81.74 |
97,0 |
-4.90 |
137,1 |
7961619 |
C.W. OF IND. STATES |
169 |
2381740 |
17.14 |
0.04 |
Africa |
Algeria |
4.61 |
6000 |
31.00 |
70,0 |
-0.39 |
78,1 |
32930091 |
NORTHERN AFRICA |
170 |
103000 |
13.64 |
4.83 |
Europe |
Iceland |
6.72 |
30900 |
3.31 |
99,9 |
2.38 |
647,7 |
299388 |
WESTERN EUROPE |
171 |
748 |
25.37 |
56.02 |
Australia |
Tonga |
5.28 |
2200 |
12.62 |
98,5 |
0.00 |
97,7 |
114689 |
OCEANIA |
172 |
27750 |
36.44 |
6.38 |
North America |
Haiti |
12.17 |
1600 |
73.45 |
52,9 |
-3.40 |
16,9 |
8308504 |
LATIN AMER. & CARIB |
173 |
1284000 |
45.73 |
0.00 |
Africa |
Chad |
16.38 |
1200 |
93.82 |
47,5 |
-0.11 |
1,3 |
9944201 |
SUB-SAHARAN AFRICA |
174 rows × 13 columns
def get_num_countries():
return countries['country'].nunique()
get_num_countries()
174
def get_total_area():
return countries['area'].sum()
get_total_area()
127170843
download('capitals.html', 'https://raw.githubusercontent.com/tylerharter/cs301-projects/master/fall19/p10/techslides-snapshot.html')
'capitals.html already exists!'
def state_stats(path):
stats = {}
f = open(path)
html_string = f.read()
f.close()
doc = BeautifulSoup(html_string, "html.parser")
trs = doc.find_all("tr")
for tr in trs[1:]:
cells = tr.find_all("td")[:4]
j = cells[0].get_text()
stats.setdefault("country",[]).append(j)
j = cells[1].get_text()
stats.setdefault("capital",[]).append(j)
j = cells[2].get_text()
stats.setdefault("latitude",[]).append(float(j))
j = cells[3].get_text()
stats.setdefault("longitude",[]).append(float(j))
return stats
wi_stats = state_stats("capitals.html")
data = DataFrame({"country":wi_stats['country'],
"capital":wi_stats['capital'],
"latitude":wi_stats['latitude'],
"longitude":wi_stats['longitude'],
})
data = data[0:]
full = pd.merge(data, countries, on=['country'], how='inner')
capitals = full[['country','capital','latitude','longitude']]
capitals.head()
|
country |
capital |
latitude |
longitude |
0 |
Afghanistan |
Kabul |
34.516667 |
69.183333 |
1 |
Albania |
Tirana |
41.316667 |
19.816667 |
2 |
Algeria |
Algiers |
36.750000 |
3.050000 |
3 |
Anguilla |
The Valley |
18.216667 |
-63.050000 |
4 |
Argentina |
Buenos Aires |
-34.583333 |
-58.666667 |
def get_country():
res = []
for i in capitals['country']:
res.append(i)
return sorted(res)
get_country()
['Afghanistan',
'Albania',
'Algeria',
'Anguilla',
'Argentina',
'Armenia',
'Aruba',
'Australia',
'Austria',
'Azerbaijan',
'Bahrain',
'Bangladesh',
'Barbados',
'Belarus',
'Belgium',
'Belize',
'Benin',
'Bermuda',
'Bhutan',
'Bolivia',
'Botswana',
'Brazil',
'Bulgaria',
'Burkina Faso',
'Burundi',
'Cambodia',
'Cameroon',
'Canada',
'Cape Verde',
'Cayman Islands',
'Chad',
'Chile',
'China',
'Colombia',
'Comoros',
'Costa Rica',
'Croatia',
'Cuba',
'Czech Republic',
'Denmark',
'Djibouti',
'Dominica',
'Dominican Republic',
'Ecuador',
'Egypt',
'El Salvador',
'Equatorial Guinea',
'Eritrea',
'Estonia',
'Ethiopia',
'Fiji',
'Finland',
'France',
'French Polynesia',
'Gabon',
'Georgia',
'Germany',
'Ghana',
'Greece',
'Grenada',
'Guam',
'Guatemala',
'Guinea',
'Guinea-Bissau',
'Guyana',
'Haiti',
'Honduras',
'Hungary',
'Iceland',
'India',
'Indonesia',
'Iran',
'Iraq',
'Ireland',
'Israel',
'Italy',
'Jamaica',
'Japan',
'Jordan',
'Kazakhstan',
'Kenya',
'Kuwait',
'Kyrgyzstan',
'Laos',
'Latvia',
'Lebanon',
'Lesotho',
'Liberia',
'Libya',
'Liechtenstein',
'Lithuania',
'Luxembourg',
'Madagascar',
'Malawi',
'Malaysia',
'Maldives',
'Mali',
'Malta',
'Marshall Islands',
'Mauritania',
'Mauritius',
'Mexico',
'Moldova',
'Monaco',
'Mongolia',
'Morocco',
'Mozambique',
'Namibia',
'Nepal',
'Netherlands',
'New Caledonia',
'New Zealand',
'Nicaragua',
'Niger',
'Nigeria',
'Norway',
'Oman',
'Pakistan',
'Palau',
'Panama',
'Papua New Guinea',
'Paraguay',
'Peru',
'Philippines',
'Poland',
'Portugal',
'Puerto Rico',
'Qatar',
'Romania',
'Russia',
'Rwanda',
'Saint Helena',
'Saint Lucia',
'Saint Vincent and the Grenadines',
'Samoa',
'San Marino',
'Saudi Arabia',
'Senegal',
'Seychelles',
'Sierra Leone',
'Singapore',
'Slovenia',
'Somalia',
'South Africa',
'Spain',
'Sri Lanka',
'Sudan',
'Suriname',
'Swaziland',
'Sweden',
'Switzerland',
'Syria',
'Taiwan',
'Tajikistan',
'Tanzania',
'Thailand',
'Togo',
'Tonga',
'Tunisia',
'Turkey',
'Turkmenistan',
'Uganda',
'Ukraine',
'United Arab Emirates',
'United Kingdom',
'United States',
'Uruguay',
'Uzbekistan',
'Vanuatu',
'Venezuela',
'Vietnam',
'Yemen',
'Zambia',
'Zimbabwe']
def get_capital():
capitals_country = capitals[capitals.country == 'Cuba']
return next(iter(capitals_country['capital']))
get_capital()
'Havana'
def get_countries():
capital = capitals[capitals.capital == 'Tbilisi']
return next(iter(capital['country']))
get_countries()
'Georgia'
def get_southern_most():
res = []
for i in capitals.sort_values(by='latitude',ascending=True)[:7]['country']:
res.append(i)
return res
get_southern_most()
['New Zealand',
'Australia',
'Uruguay',
'Argentina',
'Chile',
'Lesotho',
'Swaziland']
def get_northern_most():
res = []
for i in capitals.sort_values(by='latitude',ascending=False)[:5]['country']:
res.append(i)
return res
get_northern_most()
['Iceland', 'Finland', 'Norway', 'Estonia', 'Sweden']
df = pd.DataFrame(data = {'birth-rate':countries['birth-rate'],
'death-rate':countries['death-rate']})
df.describe()
|
birth-rate |
death-rate |
count |
174.000000 |
174.000000 |
mean |
22.463851 |
9.625172 |
std |
11.278992 |
5.187143 |
min |
8.250000 |
2.410000 |
25% |
12.597500 |
6.027500 |
50% |
20.010000 |
8.230000 |
75% |
29.860000 |
11.715000 |
max |
50.730000 |
29.740000 |
countries['literacy'] = countries['literacy'].str.replace(',', '.', regex=False)
countries['literacy'] = countries['literacy'].astype('float')
countries['phones'] = countries['phones'].str.replace(',', '.', regex=False)
countries['phones'] = countries['phones'].astype('float')
df = pd.DataFrame(data = {'literacy':countries['literacy'],
'phones':countries['phones']})
df.describe()
|
literacy |
phones |
count |
174.000000 |
174.000000 |
mean |
82.362069 |
215.770690 |
std |
20.003166 |
218.713811 |
min |
17.600000 |
1.300000 |
25% |
70.100000 |
32.000000 |
50% |
92.100000 |
140.350000 |
75% |
98.000000 |
331.750000 |
max |
100.000000 |
1035.600000 |
def get_EU_largest_land_country():
countries_EU_land = countries.loc[(countries['continent'] == 'Europe') & (countries['coastline'] == 0)]
countries_EU_land_largest = countries_EU_land.sort_values(by='area',ascending=False).iloc[0]['country']
return countries_EU_land_largest
get_EU_largest_land_country()
'Belarus'
def get_AS_largest_land_country():
countries_AS_land = countries.loc[(countries['continent'] == 'Asia') & (countries['coastline'] == 0)]
countries_AS_land_largest = countries_AS_land.sort_values(by='area',ascending=False).iloc[0]['country']
return countries_AS_land_largest
get_AS_largest_land_country()
'Kazakhstan'
def get_AS_most_pop_land_country():
countries_AS_land = countries.loc[(countries['continent'] == 'Asia') & (countries['coastline'] == 0)]
countries_AS_land_most_pop = countries_AS_land.sort_values(by='population',ascending=False).iloc[0]['country']
return countries_AS_land_most_pop
get_AS_most_pop_land_country()
'Afghanistan'
def haversine(lon1, lat1, lon2, lat2):
"""
Calculate the great circle distance between two points
on the earth (specified in decimal degrees)
"""
lon1, lat1, lon2, lat2 = map(radians, [lon1, lat1, lon2, lat2])
dlon = lon2 - lon1
dlat = lat2 - lat1
a = sin(dlat/2)**2 + cos(lat1) * cos(lat2) * sin(dlon/2)**2
c = 2 * asin(sqrt(a))
r = 3956
return c * r
Randall_lon = -89.411893
Randall_lat = 43.070231
Wisconsin_lon = -89.384113
Wisconsin_lat = 43.074645
haversine(Randall_lon, Randall_lat, Wisconsin_lon, Wisconsin_lat)
1.433899492072933
def get_distance(country1, country2):
country1_lon = capitals.loc[(capitals['country'] == country1)].iloc[0]['longitude']
country1_lat = capitals.loc[(capitals['country'] == country1)].iloc[0]['latitude']
country2_lon = capitals.loc[(capitals['country'] == country2)].iloc[0]['longitude']
country2_lat = capitals.loc[(capitals['country'] == country2)].iloc[0]['latitude']
return haversine(country1_lon, country1_lat, country2_lon, country2_lat)
get_distance('France', 'Japan')
6032.330932363535
country_list = ["Guam", "New Zealand", "Australia"]
def get_distances(country_list):
data = {}
for country1 in country_list:
tmp = []
for country2 in country_list:
if country1 == country2:
tmp.append(np.nan)
else:
tmp.append(get_distance(country1, country2))
data[country1] = tmp
df = pd.DataFrame(data, index = country_list)
return df
get_distances(country_list)
|
Guam |
New Zealand |
Australia |
Guam |
NaN |
4239.314912 |
3377.103870 |
New Zealand |
4239.314912 |
NaN |
1445.147895 |
Australia |
3377.103870 |
1445.147895 |
NaN |
def get_distance_Australian():
country_list = []
country_AU = countries.loc[(countries['continent'] == 'Australia')]
for i in country_AU['country']:
country_list.append(i)
return get_distances(country_list)
get_distance_Australian()
|
Marshall Islands |
French Polynesia |
New Zealand |
Vanuatu |
Palau |
Papua New Guinea |
Guam |
Samoa |
New Caledonia |
Australia |
Fiji |
Tonga |
Marshall Islands |
NaN |
3159.856137 |
3348.725378 |
1727.328621 |
2516.301728 |
2019.144119 |
1861.584428 |
1849.150692 |
2054.905160 |
3263.901948 |
1806.972599 |
2151.129410 |
French Polynesia |
3159.856137 |
NaN |
2668.807328 |
2765.339382 |
5447.018761 |
4265.757856 |
4961.131713 |
1496.953816 |
2864.497308 |
3920.570216 |
2102.135722 |
1686.539411 |
New Zealand |
3348.725378 |
2668.807328 |
NaN |
1671.641167 |
4218.188412 |
2767.607095 |
4239.314912 |
2062.548020 |
1400.483623 |
1445.147895 |
1613.916992 |
1510.219450 |
Vanuatu |
1727.328621 |
2765.339382 |
1671.641167 |
NaN |
2882.041892 |
1527.495923 |
2687.395771 |
1349.904435 |
335.602971 |
1687.810709 |
663.967196 |
1098.016273 |
Palau |
2516.301728 |
5447.018761 |
4218.188412 |
2882.041892 |
NaN |
1453.306058 |
800.243081 |
3956.305436 |
2974.892838 |
3099.023227 |
3468.037102 |
3931.843110 |
Papua New Guinea |
2019.144119 |
4265.757856 |
2767.607095 |
1527.495923 |
1453.306058 |
NaN |
1591.171595 |
2789.123270 |
1552.708901 |
1786.749660 |
2174.712829 |
2623.742354 |
Guam |
1861.584428 |
4961.131713 |
4239.314912 |
2687.395771 |
800.243081 |
1591.171595 |
NaN |
3520.883355 |
2871.868601 |
3377.103870 |
3165.570014 |
3617.512808 |
Samoa |
1849.150692 |
1496.953816 |
2062.548020 |
1349.904435 |
3956.305436 |
2789.123270 |
3520.883355 |
NaN |
1542.152644 |
2840.091065 |
716.316054 |
553.393188 |
New Caledonia |
2054.905160 |
2864.497308 |
1400.483623 |
335.602971 |
2974.892838 |
1552.708901 |
2871.868601 |
1542.152644 |
NaN |
1375.924712 |
825.890876 |
1179.069567 |
Australia |
3263.901948 |
3920.570216 |
1445.147895 |
1687.810709 |
3099.023227 |
1786.749660 |
3377.103870 |
2840.091065 |
1375.924712 |
NaN |
2145.768971 |
2362.766922 |
Fiji |
1806.972599 |
2102.135722 |
1613.916992 |
663.967196 |
3468.037102 |
2174.712829 |
3165.570014 |
716.316054 |
825.890876 |
2145.768971 |
NaN |
463.843872 |
Tonga |
2151.129410 |
1686.539411 |
1510.219450 |
1098.016273 |
3931.843110 |
2623.742354 |
3617.512808 |
553.393188 |
1179.069567 |
2362.766922 |
463.843872 |
NaN |
def get_most_central_AU():
df_AU = get_distance_Australian()
df_AU_mean = df_AU.mean(axis = 1, numeric_only = True)
df_AU_mean = df_AU_mean.sort_values(ascending=True)
return df_AU_mean.index[0]
get_most_central_AU()
'Vanuatu'
def get_least_central_AU():
df_AU = get_distance_Australian()
df_AU_mean = df_AU.mean(axis = 1, numeric_only = True)
df_AU_mean = df_AU_mean.sort_values(ascending=False)
return df_AU_mean.index[0]
get_least_central_AU()
'French Polynesia'
def AU_nearest_neighbor():
df_AU = get_distance_Australian()
df_AU_idxmin = df_AU.idxmin()
df_AU_min = df_AU.min()
country_list = df_AU_min.index
data = {'nearest':df_AU_idxmin.values, 'distance':df_AU_min.values}
df = pd.DataFrame(data, index = country_list)
return df
AU_nearest_neighbor()
|
nearest |
distance |
Marshall Islands |
Vanuatu |
1727.328621 |
French Polynesia |
Samoa |
1496.953816 |
New Zealand |
New Caledonia |
1400.483623 |
Vanuatu |
New Caledonia |
335.602971 |
Palau |
Guam |
800.243081 |
Papua New Guinea |
Palau |
1453.306058 |
Guam |
Palau |
800.243081 |
Samoa |
Tonga |
553.393188 |
New Caledonia |
Vanuatu |
335.602971 |
Australia |
New Caledonia |
1375.924712 |
Fiji |
Tonga |
463.843872 |
Tonga |
Fiji |
463.843872 |
def AU_furthest_neighbor():
df_AU = get_distance_Australian()
df_AU_idxmax = df_AU.idxmax()
df_AU_max = df_AU.max()
country_list = df_AU_max.index
data = {'furthest':df_AU_idxmax.values, 'distance':df_AU_max.values}
df = pd.DataFrame(data, index = country_list)
return df
AU_furthest_neighbor()
|
furthest |
distance |
Marshall Islands |
New Zealand |
3348.725378 |
French Polynesia |
Palau |
5447.018761 |
New Zealand |
Guam |
4239.314912 |
Vanuatu |
Palau |
2882.041892 |
Palau |
French Polynesia |
5447.018761 |
Papua New Guinea |
French Polynesia |
4265.757856 |
Guam |
French Polynesia |
4961.131713 |
Samoa |
Palau |
3956.305436 |
New Caledonia |
Palau |
2974.892838 |
Australia |
French Polynesia |
3920.570216 |
Fiji |
Palau |
3468.037102 |
Tonga |
Palau |
3931.843110 |
import sqlite3
conn = sqlite3.connect('countries.db')
countries.to_sql("countries", conn, if_exists="replace", index=False)
capitals.to_sql("capitals", conn, if_exists="replace", index=False)
df = pd.read_sql('select continent, count(country) AS `number of countries` from countries group by continent order by `number of countries`',conn)
df
|
continent |
number of countries |
0 |
Australia |
12 |
1 |
South America |
12 |
2 |
North America |
24 |
3 |
Asia |
38 |
4 |
Europe |
41 |
5 |
Africa |
47 |
plt.bar(df['continent'],df['number of countries'],fc = 'black')
plt.xlabel('continent')
plt.ylabel('number of countries')
plt.xticks(rotation=90)
([0, 1, 2, 3, 4, 5], <a list of 6 Text xticklabel objects>)
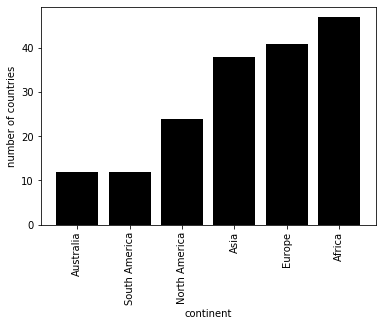
df_pop = pd.read_sql('select continent,sum(population) AS `total population` from countries group by continent order by `total population` DESC',conn)
bl = df_pop['total population']/1000000
df_pop.loc[:,'total population (million)'] = bl
df_pop.drop(columns = 'total population',inplace = True)
df_pop
|
continent |
total population (million) |
0 |
Asia |
3739.902863 |
1 |
Africa |
807.299195 |
2 |
Europe |
792.053486 |
3 |
North America |
515.041558 |
4 |
South America |
375.441666 |
5 |
Australia |
32.163025 |
plt.bar(df_pop['continent'],df_pop['total population (million)'],fc = 'black')
plt.xlabel('continent')
plt.ylabel('total population (million)')
plt.xticks(rotation=90)
([0, 1, 2, 3, 4, 5], <a list of 6 Text xticklabel objects>)
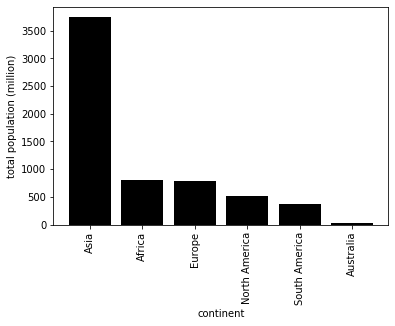
df_sou = pd.read_sql("select country,sum(population) AS population from countries where continent = 'South America' group by country order by population DESC ",conn)
df_sou
|
country |
population |
0 |
Brazil |
188078227 |
1 |
Colombia |
43593035 |
2 |
Argentina |
39921833 |
3 |
Peru |
28302603 |
4 |
Venezuela |
25730435 |
5 |
Chile |
16134219 |
6 |
Ecuador |
13547510 |
7 |
Bolivia |
8989046 |
8 |
Paraguay |
6506464 |
9 |
Uruguay |
3431932 |
10 |
Guyana |
767245 |
11 |
Suriname |
439117 |
df_top = pd.read_sql("select country,population * `gdp-per-capita` AS `real GDP` from countries order by `real GDP` DESC ",conn)
df_top_10 = df_top.head(10).copy()
bl = df_top_10['real GDP']/1000000000
df_top_10.loc[:,'real GDP (billion)'] = bl
df_top_10.drop(columns = 'real GDP',inplace = True)
df_top_10
|
country |
real GDP (billion) |
0 |
United States |
11281.191327 |
1 |
China |
6569.868565 |
2 |
Japan |
3594.473830 |
3 |
India |
3176.520785 |
4 |
Germany |
2274.855452 |
5 |
France |
1680.181354 |
6 |
United Kingdom |
1678.873538 |
7 |
Italy |
1552.164690 |
8 |
Brazil |
1429.394525 |
9 |
Russia |
1271.752506 |
ot = sum(df_top[10:]['real GDP'])/1000000000
df_top_10.loc['new'] = ['Other',ot]
df_top_10 = df_top_10.copy()
plt.bar(df_top_10['country'],df_top_10['real GDP (billion)'],fc = 'black')
plt.xlabel('contry')
plt.ylabel('GDP (billions)')
plt.ylim((0,20000))
my_y_ticks = np.arange(0, 20000,5000)
plt.yticks(my_y_ticks)
plt.xticks(rotation=90)
([0, 1, 2, 3, 4, 5, 6, 7, 8, 9, 10], <a list of 11 Text xticklabel objects>)
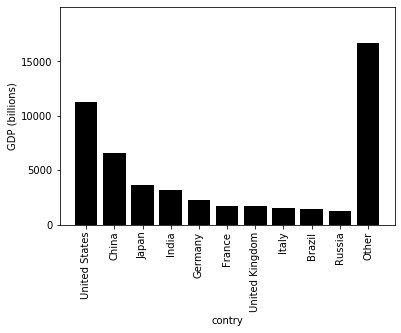
df_gap = pd.read_sql("select country,`birth-rate`,`death-rate` from countries order by (`birth-rate`-`death-rate`) DESC ",conn)
df_gap.head(15)
|
country |
birth-rate |
death-rate |
0 |
Uganda |
47.35 |
12.24 |
1 |
Yemen |
42.89 |
8.30 |
2 |
Mali |
49.82 |
16.89 |
3 |
Oman |
36.24 |
3.81 |
4 |
Madagascar |
41.41 |
11.11 |
5 |
Burkina Faso |
45.62 |
15.60 |
6 |
Niger |
50.73 |
20.91 |
7 |
Chad |
45.73 |
16.38 |
8 |
Mauritania |
40.99 |
12.16 |
9 |
Burundi |
42.22 |
13.46 |
10 |
Comoros |
36.93 |
8.20 |
11 |
Somalia |
45.13 |
16.63 |
12 |
Marshall Islands |
33.05 |
4.78 |
13 |
Maldives |
34.81 |
7.06 |
14 |
Togo |
37.01 |
9.83 |
df_gap.head(15)
ax = df_gap.head(15).plot.barh()
ax.set_xlabel("rate")
ax.set_yticklabels(df_gap.head(15).country)
[Text(0, 0, 'Uganda'),
Text(0, 0, 'Yemen'),
Text(0, 0, 'Mali'),
Text(0, 0, 'Oman'),
Text(0, 0, 'Madagascar'),
Text(0, 0, 'Burkina Faso'),
Text(0, 0, 'Niger'),
Text(0, 0, 'Chad'),
Text(0, 0, 'Mauritania'),
Text(0, 0, 'Burundi'),
Text(0, 0, 'Comoros'),
Text(0, 0, 'Somalia'),
Text(0, 0, 'Marshall Islands'),
Text(0, 0, 'Maldives'),
Text(0, 0, 'Togo')]
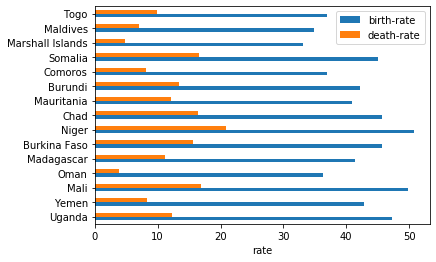
plt.rcParams["font.size"] = 10
ax = df_gap.plot.scatter(x='death-rate', y='birth-rate', c='black')
ax.set_xlabel('death-rate')
Text(0.5, 0, 'death-rate')
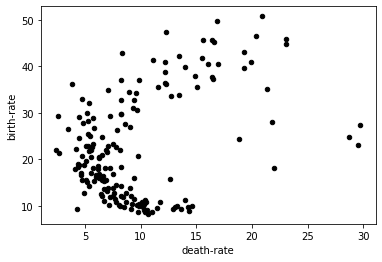
df_part = df_gap[df_gap['death-rate']>16]
df_part = df_part[df_part['birth-rate'] < 30]
df_1 = df_part[['country','death-rate','birth-rate']]
df_1.sort_values(by=['death-rate']).reset_index(drop=True)
|
country |
death-rate |
birth-rate |
0 |
Namibia |
18.86 |
24.32 |
1 |
Zimbabwe |
21.84 |
28.01 |
2 |
South Africa |
22.00 |
18.20 |
3 |
Lesotho |
28.71 |
24.75 |
4 |
Botswana |
29.50 |
23.08 |
5 |
Swaziland |
29.74 |
27.41 |
countries.corr()['death-rate']["birth-rate"]
0.3965086117752512
plt.rcParams["font.size"] = 10
ax = countries.plot.scatter(x='gdp-per-capita', y='phones', c='black')
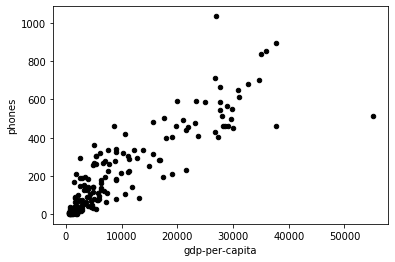
countries.corr()['phones']['gdp-per-capita']
0.8786946640591311
plt.rcParams["font.size"] = 10
ax = countries.plot.scatter(x='literacy', y='gdp-per-capita', c='black')
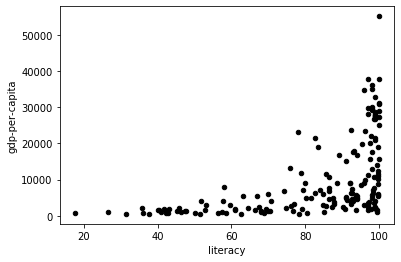
plt.rcParams["font.size"] = 10
ax = countries.plot.scatter(x='gdp-per-capita', y='death-rate',c='black')
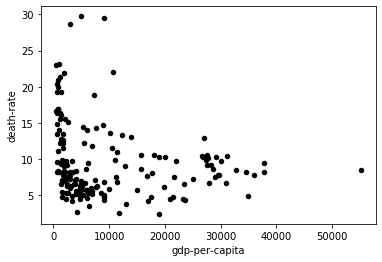
plt.rcParams["font.size"] = 10
ax = countries.plot.scatter(x='gdp-per-capita', y='birth-rate',c='black')
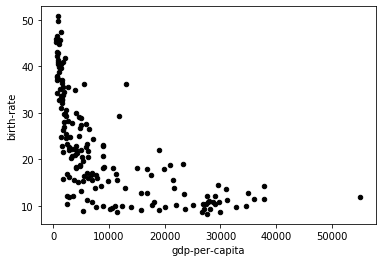
countries['inverse'] = 1/countries['birth-rate']
plt.rcParams["font.size"] = 10
ax = countries.plot.scatter(x='gdp-per-capita', y='inverse',c='black')
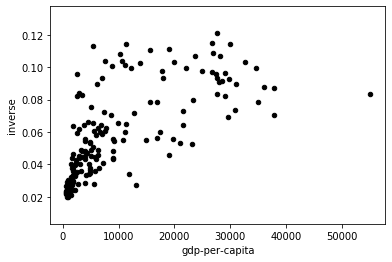
countries["1"] = 1
res = np.linalg.lstsq(countries[["gdp-per-capita", "1"]], countries["inverse"], rcond=None)
coefficients = res[0]
m = coefficients[0]
n = coefficients[1]
coef_tuple = (m,n)
coef_tuple
(1.8365703926233426e-06, 0.03916624252079354)
ax = countries.plot.scatter(x='gdp-per-capita', y='inverse', c = 'gray')
countries["fit"] = countries["gdp-per-capita"] * m + n
countries.plot.line(x='gdp-per-capita', y='fit', c='red', ax=ax)
<matplotlib.axes._subplots.AxesSubplot at 0x1a6a75308d0>
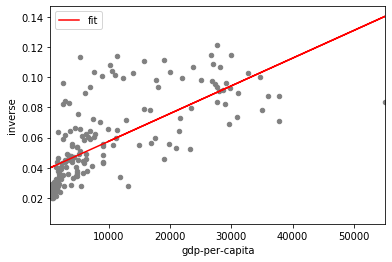
conn.close()